Last updated on April 6th, 2024 at 11:45 am
Are you on the lookout for the best machine learning courses online? Do you want to learn machine learning online but find it difficult to find the right course for you? If so, this article is for you.
In this data-centric world, learning new technologies that revolve around data is more rewarding than the conventional learning process. This is why Machine Learning, a subfield of Artificial Intelligence, is becoming popular for its ability to data processing.
Machine learning engineers build intelligent algorithms that can learn from data. This can be helpful for any industry to perform different tasks using data and build solutions for complex problems.
You can become an expert in machine learning by joining the courses available online. These will educate you about machine learning so that you can solve complex business problems using these techniques.
To make the process easier for you, we have curated this list with the 11 best machine learning courses online. Have a look and determine which course will suit your requirements.
Learn New Skills with Coursera Plus
- Coursera Plus offers education from more than 275 top colleges and businesses.
- Included in your subscription is unlimited access to over 7,000 top-notch courses, practical projects, and career-ready degree programs.
- Access videos for more than 90% of courses, professional certificates, and specializations offered by eminent faculty members from prestigious colleges and businesses.
- Take prerequisites, investigate any topic of interest or current interest, and develop your talents.
- A 7-day free trial is included with the monthly subscription, and a 14-day money-back guarantee is offered with the annual subscription. With Coursera Plus, you can access 90% of their online courses indefinitely as part of a membership plan.
- If you want to take more than one course this year, then spend less money on your education.
- You can move between courses, learn at your own pace, or choose a new course.
- Receive a certificate of completion for each course you finish at no extra charge.
- All of this for only $59 a month or $399 a year.
List Of the Best Machine Learning Courses Online
1. Machine Learning Specialization – Offered by Stanford University
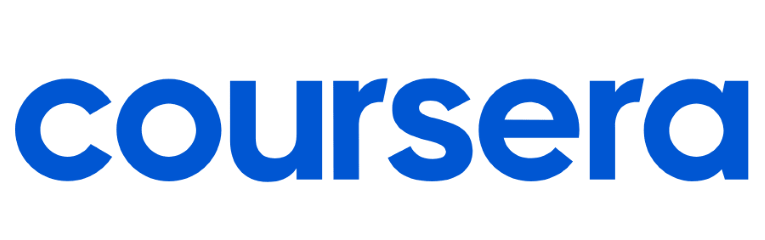
Combined with three valuable courses, the machine learning specialization is a great learning program for people who want to explore the field of machine learning. It is offered by the joint alliance of Stanford University and DeepLearning.AI.
In this specialized course, you will get a full introduction to the basics of machine learning and learn how to build AI applications using machine learning. The course includes the techniques of supervised and unsupervised learning and some effective implementation techniques for machine learning and artificial intelligence used in Silicon Valley.
Who is this course for?
This course is for anyone who wants to learn machine learning techniques and utilize them to solve complex business problems.
Students who understand computer programming with Python and possess knowledge of basic mathematics will be able to understand the concepts explained in this course.
Anyone who wants to learn the process of building machine learning models, neural networks, and different apps using machine learning can consider joining this specialization course.
Course Highlights
Institution – Stanford University, DeepLearning.AI
Platform – Coursera
Level – Beginner Level
Instructor – Andrew Ng, Geoff Ladwig, Aarti Bagul, Eddy Shyu
Duration – 2 Months
Rating – 4.9
Language Options – 21 Languages Available
Schedule Type – Flexible Scheduling
What you will learn
The machine learning specialization course begins with the basics that include popular machine libraries, i.e., NumPy and scikit-learn, supervised learning models, neural networks, decision trees, and the concepts of unsupervised learning.
You will get an overview of ML basics to creating advanced algorithms, which will pave your path of creativity and building different models using ML. Throughout this course, you will encounter 150+ video content, several reading sessions, quizzes, assignments, and applied learning projects.
The three courses included in this specialized learning program are as follows:
Supervised Machine Learning: Regression And Classification
Unsupervised Learning, Recommenders, Reinforcement Learning
2. Machine Learning Specialization – Offered by University of Washington
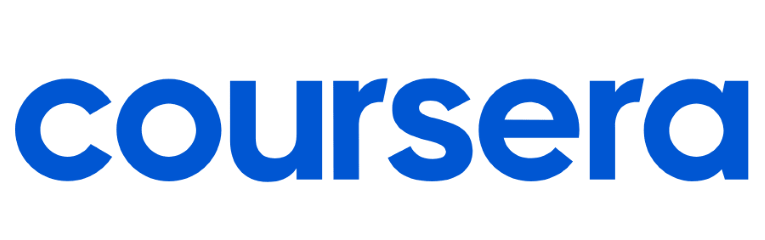
The Machine Learning Specialization course offered by the University of Washington comprises a series of four courses that give you an exciting intro to ML concepts and help build your experience in them.
In this learning program, you will learn about prediction, classification, clustering, and information retrieval in machine learning. It will teach you the analysis of complex datasets and build brilliant applications that predict using data.
Who is this course for?
This specialization course is an intermediate-level course. Hence, candidates should have some experience in a related field to understand the topics explained in this course. Specifically, having experience in Python programming will be helpful for you in this course.
Anyone who wants to master the fundamentals of machine learning and build smart applications using them can consider enrolling in this specialized course.
Course Highlights
Institution – University of Washington
Platform – Coursera
Level – Intermediate Level
Instructor – Emily Fox, Carlos Guestrin
Duration – 2 Months
Rating – 4.7
Language Options – 22 Languages Available
Schedule Type – Flexible Scheduling
What you will learn
Out of four courses in this specialization course, the first course discusses regression, classification, clustering, recommender systems, and deep learning. It also explains how to build ML models using data as input and build an application using ML finally.
The second course is about regression in machine learning. You will learn everything about the concept of regression in this part. The 3rd and 4th courses discuss the concepts of classification, Clustering, and Retrieval.
In each course, you will build an applied learning project using your learnings. The four courses included in this specialized program are as follows:
Machine Learning Foundations: A Case Study Approach
Machine Learning: Classification
Machine Learning: Clustering And Retrieval
3. Deep Learning Specialization – Offered by DeepLearning.AI
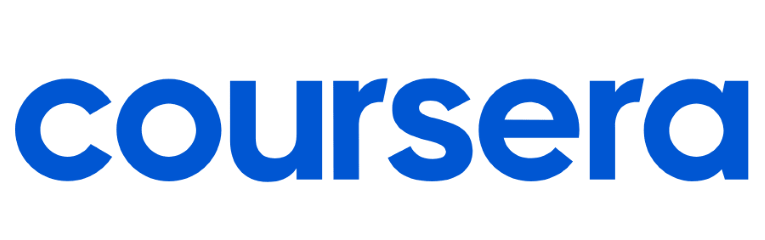
The Deep Learning Specialization course is a fundamental course that discusses the concepts of deep learning. You will encounter the abilities, challenges, and outcomes of deep learning throughout this course.
Topics like convolutional neural networks, recurrent neural networks, transformers, and LSTMs are covered in this course, which will help you in the field of AI. This specialization course acts as a pave for anyone who wants to build a career in the field of AI, providing you with the knowledge and skills you need for your goal.
Who is this course for?
Anyone who is in the field of machine learning and AI and wants to learn more about neural networks and deep learning to build AI applications can enroll in this specialized course.
Since this is an intermediate-level course, having basic knowledge of algebra, machine learning, and Python programming skills such as loops, conditional statements, and data structures will help you grasp the topics explained in this course better.
If you are someone who wants to explore the field of deep learning and understand its functions from the back end, then this course is for you.
Course Highlights
Institution – DeepLearning.AI
Platform – Coursera
Level – Intermediate Level
Instructor – Andrew Ng, Younes Bensouda Mourri, Kian Katanforoosh
Duration – 3 Months
Rating – 4.9
Language Options – 22 Languages Available
Schedule Type – Flexible Scheduling
What you will learn
You will learn the basics of neural networks, from building to training and applying them in your projects. You will have an idea of deep learning and how it will help you in the development of AI.
The second part of this course goes a step deeper, enabling you to build deep learning applications and use different techniques like hyperparameter tuning, regularization, and optimization.
The next sessions of this course discuss machine learning projects, convolutional neural networks, and sequence models. By the end of this course, you will have a deeper understanding of neural networks and deep learning concepts and be able to build projects using machine learning algorithms.
4. Introduction To Machine Learning with TensorFlow – Offered by Udacity platform
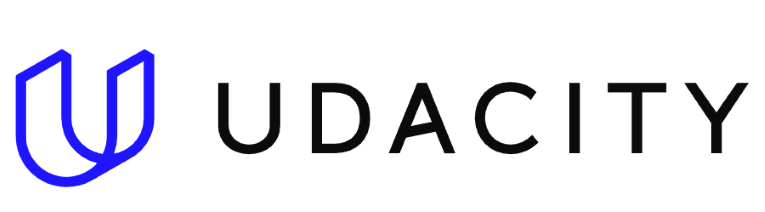
Introduction To Machine Learning with TensorFlow is an excellent course on the Udacity platform that contains four courses inside. It introduces machine learning fundamentals using the TensorFlow software library.
Students will learn supervised learning, unsupervised learning, and neural networks in this course. Each course is divided into multiple lessons covering different topics, allowing you to understand each topic thoroughly.
Who is this course for?
This is an intermediate course designed for students who are willing to build a career in the field of machine learning and artificial intelligence. This course requires a basic understanding of mathematics, python programming, and supervised machine learning to understand the topics better.
If you want to learn the basics of neural networks with the TensorFlow software library, then this course is for you.
Course Highlights
Platform – Udacity
Level – Intermediate Level
Instructor – Josh Bernhard, Michael Virgo, Mat Leonard, Andrew Paster, Jennifer Staab, Dan Romuald Mbanga, Cezanne Camacho, Sean Carrell, Jay Alammar, Luis Serrano, Juan Delgado
Duration – 2 Months
What you will learn
The first course gives a brief introduction to the topics you will learn. Also, it teaches how to set up Python 3 on your computer. Then, the course will cover the concepts of supervised learning, its types, and how to use them to solve complex problems.
It includes different topics such as Linear Regression, Perceptron Algorithm, Decision Trees, Naive Bayes, Support Vector Machines, Ensemble Methods, Model Evolution Metrics, and Training and Tuning. The final lesson covers building a project using methods you have learned in this course.
The 3rd course covers the basics of neural networks and deep learning. You will learn how to implement neural networks using Python and TensorFlow. The final course covers topics related to unsupervised learning and how to apply them to solve complex problems.
5. Introduction To Machine Learning – Offered by Udacity
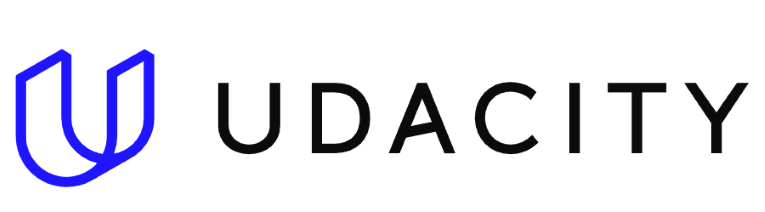
This machine learning course is designed with an alliance between AWS and Udacity to let you learn high-level concepts of machine learning through AWS SageMaker.
You will learn what SageMaker Studio is and use this platform for data analysis. This course will give you an idea about determining the perfect scenario to apply machine learning concepts to solve real-world problems. Also, you will be introduced to modern ML models such as XGBoost and AutoGluon.
Who is this course for?
This course is the perfect choice for anyone who is interested in exploring the field of machine learning or wants to explore the AWS SageMaker machine learning platform. People who are in the field of machine learning and innovation can consider joining this course to understand upgraded concepts.
Pupils who want to join this course should know basic Python, intermediate Python, Pandas, Jupyter Notebooks, and basic probability. Knowing these will help you understand the topics explained in this course.
Course Highlights
Platform – Udacity
Level – Intermediate Level
Instructor – Matt Maybeno
Duration – 4 Weeks
What you will learn
This introductory course on machine learning includes six lessons, out of which the first one gives a brief intro about machine learning and the topics you are going to learn on this course.
Then, you will learn what AWS SageMaker Studio is and how to perform exploratory data analysis on this platform. The next lessons will teach you different concepts of machine learning, tools, and algorithms, enabling you to use the AWS SageMaker platform effectively.
In the final session, you will create a learning project applying your skills and learnings throughout this course.
6. Machine Learning with Python – Offered by FreeCodeCamp
Machine Learning with Python is a free course offered by FreeCodeCamp that teaches you to apply machine learning concepts in your projects. This course uses the TensorFlow platform to create neural networks and understand the techniques of natural language processing and reinforcement learning.
Also, this course gives an intro to different types of neural networks, such as deep, recurrent, and convolutional. By the end of this machine learning course, you will have an idea about what machine learning is and how to build algorithms and models using machine learning techniques.
Who is this course for?
If you are planning to learn machine learning and build several applications using TensorFlow and Python, then this course will be the best choice for you. This course is available for free, so anyone who is interested in this field can consider enrolling in this course.
It contains the fundamentals of TensorFlow, the working of neural networks, and projects related to machine learning with Python. If you want to learn these techniques, consider joining this course.
In addition to that, having knowledge in the field of mathematics and Python programming will enable you to grasp the concepts in this course better.
What you will learn
The first part of this course discusses what the TensorFlow framework is and how it makes the implementation of neural networks easier. It covers topics from introduction to algorithms and different types of neural networks.
The next chapter explains how neural networks work, which is designed specifically for beginners. It explains the concepts in such a way that even those who are new to this field can easily understand them.
Then, you will build several projects using machine learning and Python. In this process, you will apply all your skills and learnings of machine learning.
7. Machine Learning for All – Offered by the University of London
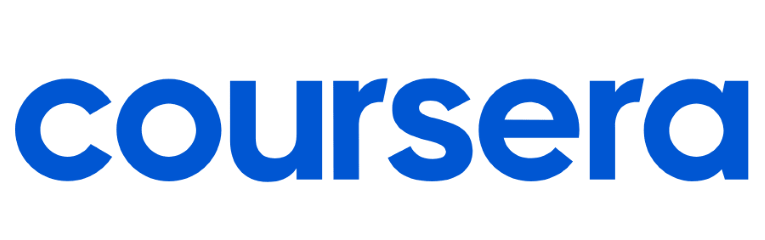
Machine Learning for All is an introductory machine learning course by the University of London. This course discusses the workings of modern machine learning technologies and how to predict results using data.
This is a non-technical course, so it doesn’t have any programming aspects such as Python or TensorFlow. It covers the concepts of machine learning without programming, enabling everyone to learn this buzzing technology.
Who is this course for?
Since this course explains the topics of machine learning without needing the learners to have experience in programming, anyone who wants to learn machine learning without any programming knowledge can join this course.
This course can be a medium for many who want to explore ML systems without going deeper into technical details. It is also suitable for those who are in a non-technical job role and want to learn what machine learning is.
Even if you just have some interest in machine learning and want to explore this field, this course will be the right choice.
Course Highlights
Institution – University of London
Platform – Coursera
Level – Beginner Level
Instructor – Prof Marco Gillies
Duration – 21 Hours
Rating – 4.7
Language Options – 22 Languages Available
Schedule Type – Flexible Scheduling
What you will learn
The first module of this course discusses machine learning and artificial intelligence techniques and how these techniques address practical problems. This course explains what data features are and how they make the learning process easier.
The next module of this course is building your own machine learning project and applying your learnings to ensure that it works as desired. You will have to perform tasks like collecting a dataset, training a model, and testing a model in this project.
By the end of this course, you will have an appropriate understanding of machine learning and can identify its benefits and dangers for society.
8. Machine Learning with Python – Offered by IBM
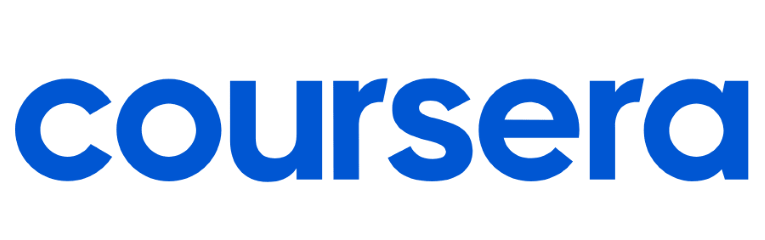
Machine Learning with Python by IBM is perfect for you if you want to learn different types of machine learning algorithms and their applications. Those who want to start their career in machine learning can consider studying this course.
This course describes supervised and unsupervised learning, classification techniques, linear and non-linear regression, and many other concepts used in machine learning. This course will make you job-ready or help advance your career in the data science field.
This machine learning course is a part of two professional certificate courses offered by IBM – IBM AI Engineering Professional Certificate and IBM Data Science Professional Certificate.
Who is this course for?
This course is a perfect choice for those who want to explore the field of ML using Python. It will help them understand what machine learning is and the different components of it.
If you are in a job position in a similar field and want to learn machine learning due to the demand of the job, this course can be a great choice to start with. For those who want to learn machine learning with IBM, this course will be great for them.
Course Highlights
Institution – IBM
Platform – Coursera
Level – Intermeiate Level
Instructor – Saeed Aghabozorgi, Joseph Santarcangelo
Duration – 12 hours
Rating – 4.7
Language Options – 22 Languages Available
Schedule Type – Flexible Scheduling
What you will learn
The first chapter gives an overview of machine learning, describing its concepts and applications. You will understand what supervised and unsupervised machine learning are and how to use them in different applications.
The next chapter describes different types of regression methods, such as linear, non-linear, simple, and multiple regression, and their applications. Then, you will get an intro about classification algorithms and their advantages and disadvantages.
Then, there will be a chapter on clustering algorithms and their uses. Finally, there will be a project in which you have to implement everything you have learned throughout this course. After completing the project, you can submit the report for peer assessment.
9. Machine Learning DevOps Engineer – Offered by Udacity
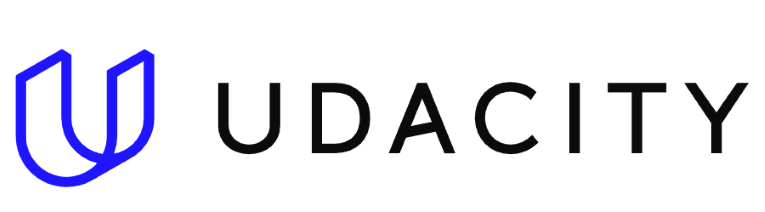
Machine Learning DevOps Engineer is a nano degree program that explains how to employ data and machine learning models in a production environment using the fundamentals of software engineering.
This course will develop DevOps skills in students that will help them automate machine learning models. It is a 4-month advanced-level program that requires students to understand Python, data science, machine learning fundamentals, Python Libraries, and APIs.
Who is this course for?
Anyone who is looking to advance their skills in machine learning and develop DevOps skills can consider joining this course. If you want to learn how to automate different stages and aspects of machine learning, you can learn this by joining this course.
This machine learning course is also suitable for those who are working in the field of machine learning and want to implement aspects of software engineering in machine learning can consider enrolling in this course.
To understand the topics explained in this course, students need knowledge of basic to intermediate Python, data science, machine learning fundamentals, basic descriptive statistics, and RESET APIs.
Course Highlights
Platform – Udacity
Level – Advanced Level
Instructor – Giacomo Vianello, Ulrika Jagare, Justin Clifford Smith, Bradford Tuckfield, Joshua Bernhard
Duration – 4 months
What you will learn
First, you will get an intro to the Machine Learning DevOps Engineer nano degree program and come to know about your journey through the lessons of this course. This chapter will brief everything that you are going to learn in this course.
In the next chapter, you will learn skills to develop machine learning modes for a production environment. This includes coding best practices using PyLint and AutoPEP8 and advanced skills in Git and GitHub.
Then, you will learn modern and real-world machine learning projects using reproducible workflows. You will know the basics of MLops and perform various tasks using it. Also, it covers Kubernetes, Kubeflow, and Great Expectations technologies.
The next chapter discusses ML Model scoring and monitoring and includes topics like model deployment and model drift. It also includes problem-solving skills to enable you to diagnose and fix operational problems.
Then, you will learn how to deploy a scalable machine learning pipeline in production and all aspects regarding this. With this, the course ends, and you will get a nanodegree certificate.
The final session of this course will teach you how to improve your LinkedIn profile and optimize your GitHub profile to increase your chances of getting a job.
10. Mathematics For Machine Learning Specialization – Offered by Imperial College London
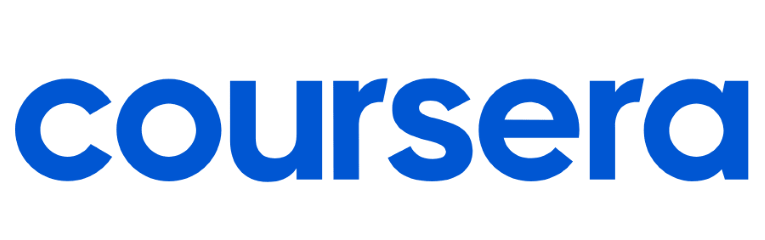
When it comes to learning machine learning or data science, students need mathematics basics as a prerequisite. Even if you have studied basic mathematics in school, it can be difficult to put those learnings into this context.
So, to freshen up your mathematics knowledge and help you resonate with the mathematical topics in the field of computer science, this course is designed.
Designed by Imperial College London, this specialized course on mathematics aims to revise your mathematical learnings and explains how to relate mathematics to ML and data science.
Who is this course for?
Anyone who finds it difficult to understand topics in machine learning or data science due to mathematical terms should join this course.
If you can’t resonate mathematics with the concepts of machine learning and data science, then this course will be the right choice for you.
For those who want to join a revision course on mathematics before joining a machine learning course, this course will be the best one to choose.
Course Highlights
Institution – Imperial College London
Platform – Coursera
Level – Beginner Level
Instructor – David Dye, Samuel J. Cooper, Marc Peter Deisenroth, A. Freddie Page
Duration – 1 Month
Rating – 4.6
Language Options – 22 Languages Available
Schedule Type – Flexible Scheduling
What you will learn
The first course of the Mathematics for Machine Learning Specialization program is linear algebra. You will learn the basics of linear algebra and how to use it for data. It also covers vectors and matrices and how to use them.
The next course is about Multivariate Calculus, which plays a crucial role in building machine learning techniques. First, you will be introduced to this topic. Then, you will use vectors and matrices with data. Also, it shows how calculus is applied in neural networks and linear regression models.
The final course is about Principal Component Analysis (PCA), which teaches how to compress high-dimensional data using the topics you have learned from the previous two courses. This one is an intermediate-level course, so you need to know NumPy and Python to understand the concepts.
11. Machine Learning Engineer with Microsoft Azure – Offered by Udacity
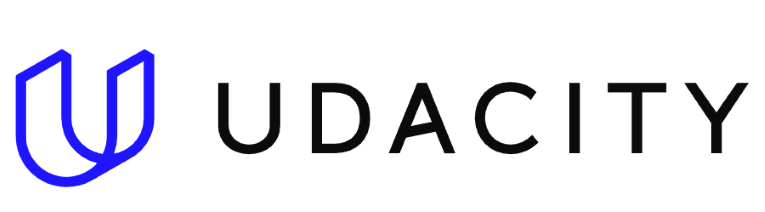
This is a nano degree program that uses MLOps of Microsoft Azure to explain Machine Learning Models to students. It also discusses data protection and different aspects of Azure Machine Learning.
By the end of this course, you will learn how to use Azure Machine Learning and be able to perform different machine learning operations. In the end, there will be a project on problem-solving, which you have to do using your learnings of Azure Machine Learning.
Who is this course for?
Anyone who is looking to learn Microsoft Azure and its role in machine learning can join this course.
If you are in a job that requires you to gain skills in machine learning and the Azure platform, this course will be the right choice to start with. In addition to the Azure platform, this course also covers machine learning operations such as the deployment of models, pipeline automation, etc.
This makes this course suitable for anyone who wants to learn different operations in machine learning.
Course Highlights
Platform – Udacity
Level – Intermediate Level
Instructor – Erick Galinkin, Noah Gift, Soham Chatterjee, Alfredo Deza
Duration – 3 Months
What you will learn
The beginning of this course gives a brief introduction to the Machine Learning Engineer program with Microsoft Azure. It will explain the structure of the course and introduce the instructors of this course.
The second part is all about the Azure platform and how to build machine learning pipelines using Azure. Also, you will learn to build automated ML models using the Azure ML SDK.
Then, the course covers the operations of machine learning and all concepts related to the same. Finally, you will build a capstone project applying all your learnings of the Azure Machine Learning nano degree program to solve a problem.
FAQ
What is machine learning?
The development of techniques for computers to learn and perform better is the main goal of machine learning (ML), a subfield of computer science and artificial intelligence (AI).
Machine learning (ML) teaches computers by giving them problems to solve and a plethora of instances (combinations of problem-solution) to model from rather than by presenting them with exact instructions through programming.
A large collection of labeled images can be used to teach a computer, for example, to recognize images of trucks and kittens. This training enables the computer to identify essential characteristics that differentiate a vehicle from a cat. The computer can identify trucks and cats in pictures it has never seen before once it has been trained.
In what way might machine learning be applied?
The steps involved in machine learning have been delineated here.
Step 1: Compiling The Data
Obtaining data from several sources and organizing it into training data for use in the subsequent step is the first phase in the data gathering process.
Step 2: Teaching The Machine
To help the computer recognize patterns in the data, this stage involves using the training data to train the computer.
Step 3: Building The Model
From the learned data, the computer may now produce models that are used to classify data or make specific predictions.
Step 4: Model Testing and Assessment
Using several sets of data, the method verifies the accuracy of the constructed models.
Step 5 – Deployment
Once the testing and evaluation process of the created models is done, these models become available for real-world applications.
Which machine learning model types exist?
Several categories of machine learning models exist, including:
Under supervised learning, patterns and predictions are learned using labeled data (input-output pairs).
Analyzing unlabeled data to find hidden patterns or groupings is known as unsupervised learning.
Semi Supervised learning: Merges annotated and unannotated data.
Reward or penalty-based decision-making is taught to models through reinforcement learning.
What characterizes deep learning as opposed to machine learning?
Numerous methods for teaching computers to learn are included in the larger area of machine learning.
Multiple-layer neural networks, or deep neural networks, are the exclusive focus of deep learning, a type of machine learning. Natural language processing and picture identification are two areas where deep learning has shown impressive promise.
What distinguishes regression from classification in machine learning?
Regression forecasts continuous numerical quantities, such as home values.
Data is classified into specified categories (spam or not spam, for example) in classification.
A neural network: what is it?
An ML model modeled like the human brain is called a neural network. It is made up of layers of networked nodes or neurons. Multiple hidden layer deep neural networks are used in deep learning.
Conclusion
You have just experienced the 11 best machine learning courses available online, and it is now up to you which course you want to select for your learning. Meanwhile, you can view our article featuring the Best Python Courses for Beginners. These courses will help you understand the concepts of Python, which will be helpful while studying machine learning courses.
If you are in the field of web development or app development, then our articles on Best Java Courses and Best JavaScript Courses will be helpful for you.
Related Articles
Best Python Courses for Beginners
Deep Learning Vs Machine Learning: What is The Difference?
Discover more from Technical Studies
Subscribe to get the latest posts sent to your email.